Getting testy
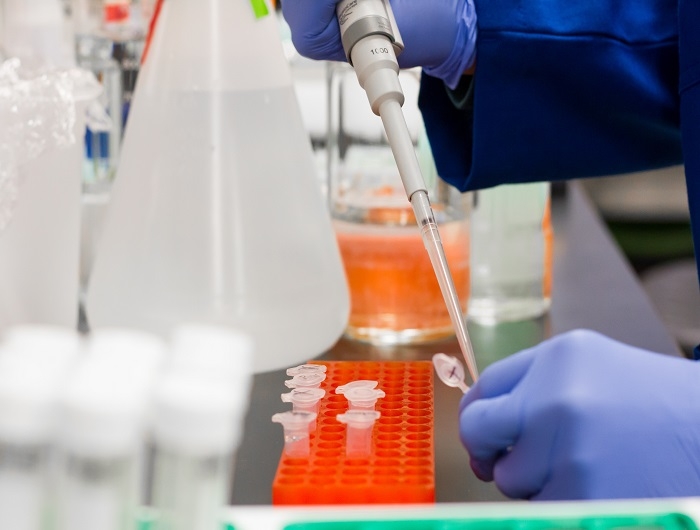
Beyond the curve: Dr. Peter Lurie's COVID-19 blog
See all Beyond the Curve posts
OK, I admit it. This blog has gotten pretty political. It’s hard to avoid, in a pandemic that has been politicized from the get-go.
Time, then, to go full-on nerd. In this blog, I’ll talk about antibody testing, how to interpret results, and the dangers of inaccurate tests. I addressed the regulatory aspects of these tests in an earlier blog post.
First, some background. Viral tests have received most of the ink so far in this pandemic; they measure whether the coronavirus is actually present. Antibody tests, on the other hand, are about the body’s reaction to the virus; an antibody is a chemical produced by the body in its efforts to fight off an infection. Certain antibodies stick around even after the illness is over and therefore can be used to measure previous infection. For many viruses, the presence of antibodies signifies that the body has successfully fought off the infection, and the person is now immune from reinfection.
So, an antibody test has several general uses. (It’s not very useful in treating an acutely sick person; in that situation, a viral test is more helpful.) First, it can be used by public health authorities to assess population levels of immunity. Second, it could be used to identify people who are immune and who can then return to work or otherwise be exposed, say in caring for loved ones. Third, the antibodies themselves could be harvested, as some have suggested that convalescent plasma could be a treatment for COVID-19.
Unfortunately, though, much remains unknown. We still don’t have proof that the antibodies are protective against the coronavirus at all and, even if they are, we don’t know how long that protection will last. And there are problems with the interpretation of the tests themselves. Buckle your seatbelts! Here’s the technical bit.
The first and most important thing to understand is that all tests inevitably make at least some errors. So there can be a difference between what the test result is and what is actually true in the world. As the names imply, true positives and negatives are tests in which the result, whether positive or negative, accurately reflects reality. A false negative, however, occurs when someone has the disease, but the test says they don’t; conversely, a false positive occurs when someone does not have the disease, but the test says they do.
Depending on the circumstances, either mistake can be critical. For example, a false negative test for a fatal infection with an available cure would be tragic. And a false positive test for a cancer that then leads to unnecessary surgery would be likewise be awful. You can see the four possible outcomes in this table:
Patient Tests Positive | Patient Tests Negative | |
Patient has the Disease | True positive | False negative |
Patient does not have the Disease | False positive | True negative |
You’ve probably also seen the terms “sensitivity” and “specificity” bandied about. They can be understood directly from the table. Sensitivity is the probability that a test detects disease if it is present. Now, the number of people with the disease is the sum of the true positives and the false negatives (first row). So, in mathematical terms, sensitivity is defined as (true positives) ÷ (true positives + false negatives). Slightly harder to wrap your head around is the specificity – the fraction of people without the disease who have a negative test. The number of people without the disease is the sum of the false positives and the true negatives (second row). And specificity is defined as (true negatives) ÷ (false positives + true negatives).
With me so far? The problem is that after being tested all you have is the result; you may not have what is actually true. So the question becomes: what is the probability that the test result you have is actually true?
Let’s first consider a positive test. You’d want to look at all the positives (true positives + false positives; column 1). The fraction of all positive tests that are accurate is (true positives) ÷ (true positives + false positives). This has a clumsy, but appropriate name: the positive predictive value, because it tells you how likely your positive test is to be right. Conversely, the negative predictive value is the fraction of all negative tests (column 2) that are correct: (true negatives) ÷ (true negatives + false negatives).
OK, that’s it with the nomenclature, unless you’re not sure what “prevalence” means (many don’t). In epidemiology, prevalence is the fraction of the population being tested that really has the disease. (If you’ve come to love the table, it’s (true positives + false negatives [row 1]) ÷ (the sum of all 4 cells). And prevalence matters because while, generally speaking, sensitivity and specificity do not vary much with prevalence, positive and negative predictive values do. And, to get us back to where we started, that will have a lot to do with whether an antibody test performs well in practice.
Turns out the performance characteristics of many of the antibody tests on the market are not that great. (As noted in my companion blog, we have the FDA in part to thank for that.) My colleague Deborah Zarin from Harvard, who provides an even more detailed take on diagnostic testing here, estimates the sensitivity and specificity of the antibody tests at 95% each (probably better than some of the poor tests that have been marketed to date). Sounds pretty good, right? Not so fast.
Let’s go back to our trusty table, and first consider a relatively high prevalence situation. (I’ve changed “disease” to “antibodies” because now we’re testing for the presence of antibodies, not actual infection.) In the United States, probably the highest antibody prevalence has been about 20%, in New York City. So for every 100 people, 20 have the condition (true positives + false negatives) and 80 do not. We show that in the table as follows:
Patient Tests Positive | Patient Tests Negative | Total | |
Patient has Antibodies | True positive | False negative | 20 |
Patient does not have Antibodies | False positive | True negative | 80 |
Now, the sensitivity of the test is 95%, which means that 95% of the 20 people or 19 people will test positive and you’ll miss one. Similarly, the specificity of the test is 95% which means that 95% of the 80 people without the disease will test negative (76 people). So now our table looks like this:
Patient Tests Positive | Patient Tests Negative | Total | |
Patient has Antibodies | 19 | 1 | 20 |
Patient does not have Antibodies | 4 | 76 | 80 |
Total | 23 | 77 |
Nearly there! So, now you have 23 positive tests and 19 of them (83%) are true. That’s right – it’s the positive predictive value. And the negative predictive value is 76/77 or 99%. So if you have a negative test, you very likely don’t have antibodies; if you test positive, there’s a 17% chance that you really don’t have them. Not great.
But it gets worse. Now let’s say the prevalence is actually 5%, more like what you’ll find in the rest of the U.S., other than heavily affected urban centers. Let’s fill the table out again, with the same test characteristics, but with 5 people having antibodies and 95 not having them. After rounding, five people have antibodies and all test positive because the sensitivity is 95%. And, with a specificity of 95%, 90 of 95 people test negative.
Patient Tests Positive | Patient Tests Negative | Total | |
Patient has Antibodies | 5 | 0 | 5 |
Patient does not have Antibodies | 5 | 90 | 95 |
Total | 10 | 90 |
At this lower prevalence, a negative test is even more predictive: If you test negative, you definitely don’t have antibodies. But if you test positive, as 10 people will, your positive predictive value is only 5 ÷ 10 or 50%. Your positive test is as likely to be wrong as right. And if the prevalence is still lower, as is likely true in many parts of the U.S., your positive test is most likely wrong!
What are the implications of this? First, a negative result is likely to be accurate. Second, a positive result may well not be, especially if few people truly have antibodies. And the danger of the low positive predictive value is that people might use the result to relax social distancing, potentially exposing themselves or others. Belatedly, FDA seems to have come to this realization and is now requiring stronger regulation of these tests. But how many people have already been misinformed?
Tags
Topics
Contact Info: Contact us at cspinews[at]cspinet.org with questions, ideas, or suggested topics.